Published: 8 April 2020 14:00
When on March 31 we started publishing about our forecasting scenarios of the COVID-19 epidemic in Belgium, we did so with the data provided by Sciensano, the Belgian Federal Health Agency. From the start of the epidemic onwards, they had been collecting the data about hospitalisations, ICU admissions, deaths etc. Our models rely on their time series from early March onwards.
At first we only based our work on the official data as announced during the daily press briefing, and we allocated the reported data to the previous day. So data that was reported on March 31 (reported observations), we allocated to March 30 (allocated observations) to run our model.
This allocation was only partially correct, at least for the deceased.
Sciensano provided us with the actual data from April 1 onwards. It turned out that the reported numbers sometimes concerned deaths of more than 1 week before.
Testing for robustness
No registration system is perfect; therefore we also frequently test the robustness of our predictions for missing data. We do this by exploring what happens if we are missing 5 to 15% of the real observations… Our findings were re-assuring. Such deviations only had a minor impact weeks later: the peak would be a bit higher (e.g. 160 versus 140) and a few days later.
With the new series of allocated observations for the deceased, we reran the model, updated our predictions, and updated our initial web report with the following note PS: What if the underlying data are not accurate.
Our models still confirmed the peak of this epidemic was near. From Saturday April 4 the decline would set in with end states ranging from 1.800 to 2.100 casualties.
In fact, we even stopped making forecasts for the ICU capacity on April 7, as it was clear that Belgian ICU capacity was in the safe zone and our models have predicted the peak and plateau accurately. (Later this week we will publish here about this part of our work.)
Incomplete data from retirement homes
On April 7 it became clear that the registrations of people who had died related to COVID-19 in retirement homes, had not been completely and correctly integrated in the data provided. An additional number was reported and this time it had a serious impact on our forecasts. (Right now, we are still not sure about more than 600 deaths in the retirement homes, whether they have already been reported upon or not).
When time series are incomplete up to 50% or more - as is the case - forecasts tend to lose their relevance/predictive validity. The situation becomes even worse if the incompleteness of the data displays characteristics of a random walk.
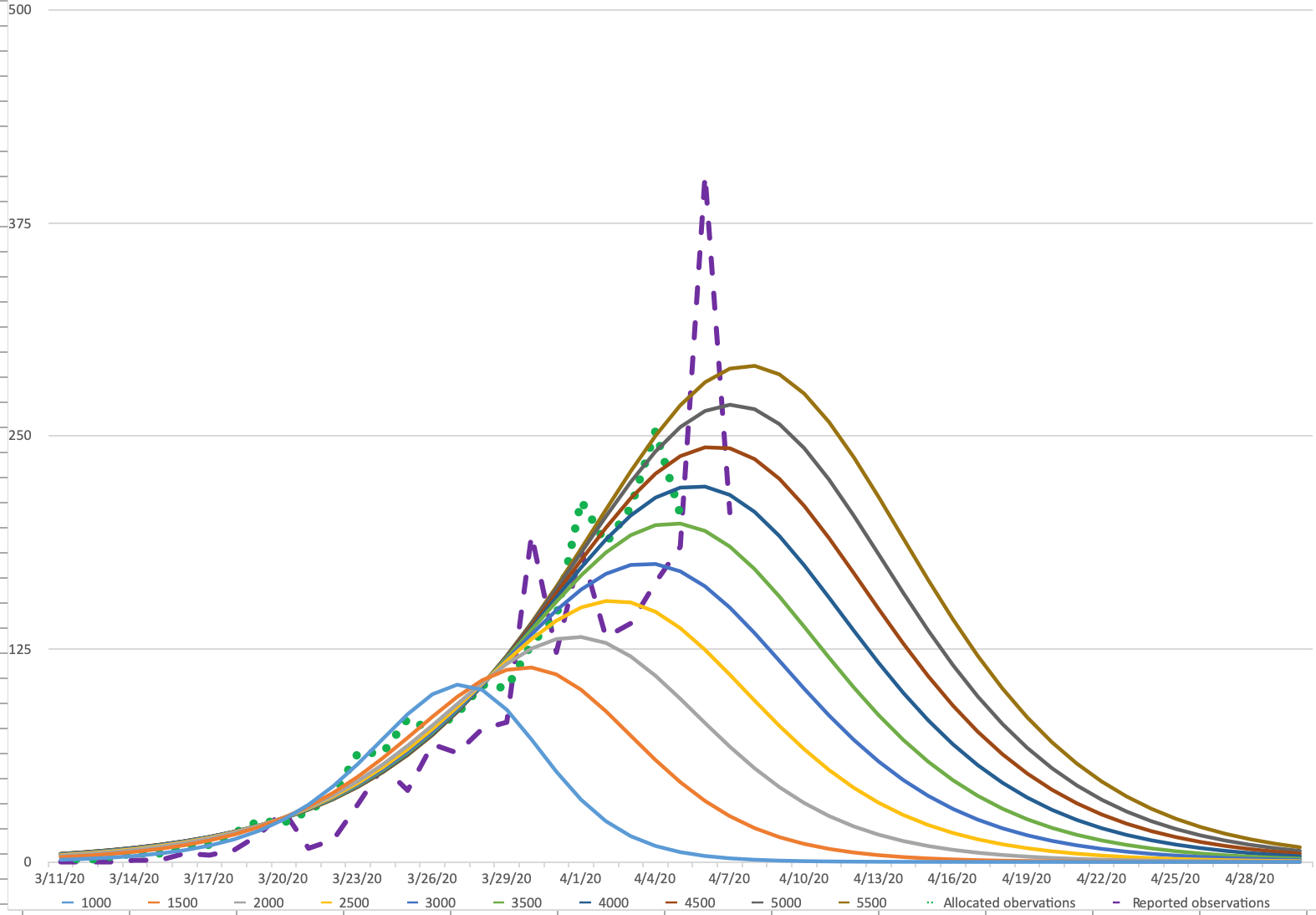
In this figure you see the reported observations and the allocated observations (actual day of death).
In this situation, no single forecast (whichever method is being used) will be relevant or accurate.
The design of the model shows the landscape
So if the data are flawed, how do we know that the model works?
By design, our scenario-driven forecasting approach enables high end states to reveal itself. Also for Belgium, our model flagged potential end states amounting to 10.000 casualties during our first runs which we started on 20 March. They were just more unlikely, based on the initial data we received.
The higher end states we face now don’t come as a surprise to us.
However, the unfolding pathways change abruptly, and this has implications: the decline sets in later, the end state will be higher.
We are aware that increasing the end states creates discomfort and unease. As such a plea for more accurate and timely available data remains warranted.
Coming soon
We have also applied our model to other countries. Soon you will read here of the forecasts we made for Italy.
Also, we are wrapping up two papers to be published in the coming days, as soon as they are available, you will find links here as well.
In the meantime we highly recommend the paper from Robert A. Burgelman & Andrew S. Grove: Let Chaos Reign, then rein in chaos.